Taking the right look at the search queries of your campaigns offers a very high potential for optimizing your performance? We have approaches to analyze n-grams of user queries and map all relevant KPIs like Cost Per Order, Conversion Rates, Value Per Click, etc.
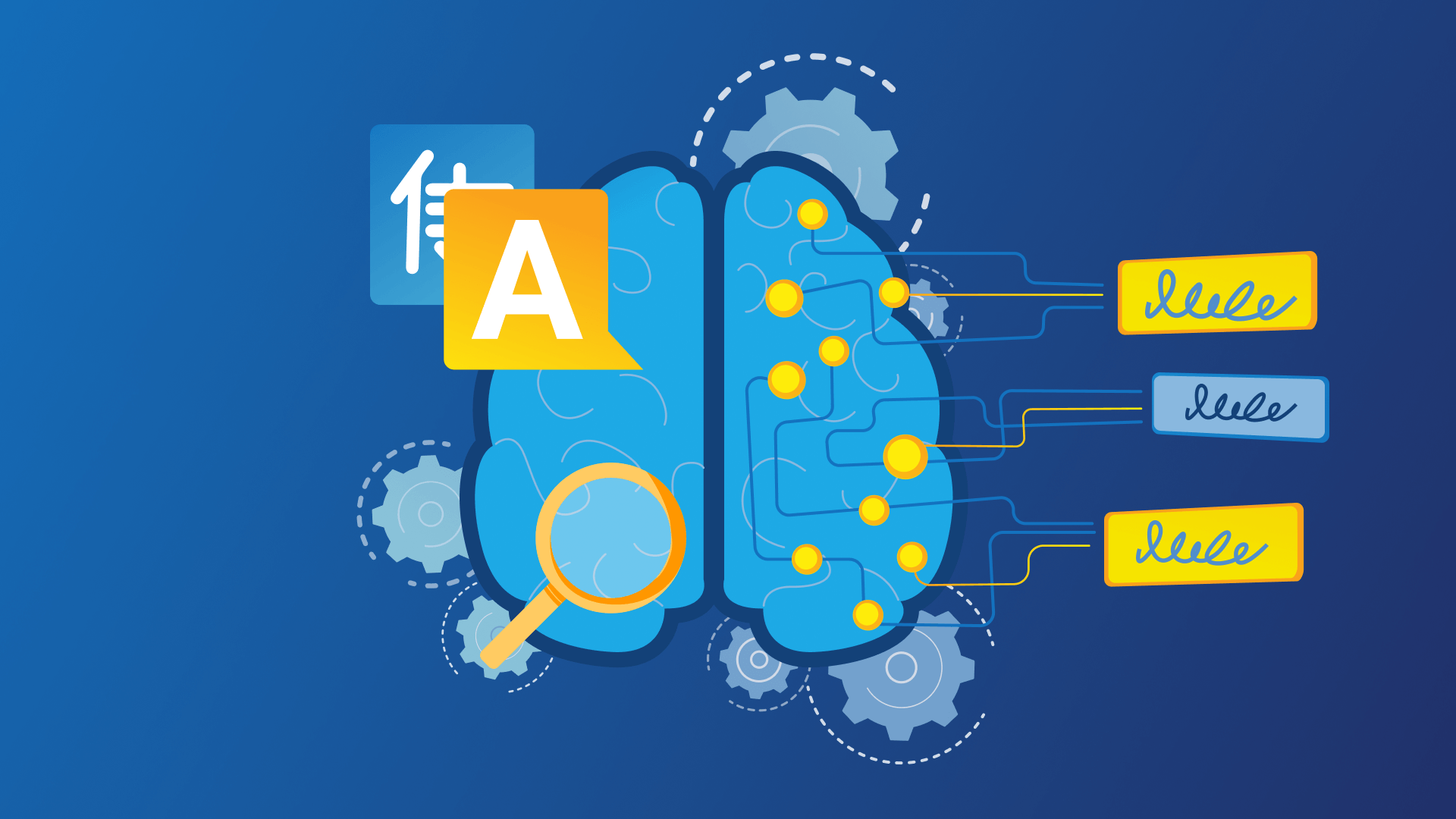
What is n-gram?
Let’s explain this with an example. “Hugo boss onlineshop” is our search query.
1-grams would be: | 2-grams would be: |
---|---|
hugo | hugo boss |
boss | hugo onlineshop |
onlineshop | boss onlineshop |
If you do this across thousands of queries, you’ll find interesting patterns that perform differently. Suppose performance is poor. Then you should add some negative keywords for that pattern.
This approach works quite well if you have a sufficient sample size (like clicks > 100) for an n-gram pattern. The problem is that there are still many rare words that you waste a lot of money on. However, it would take too long to check them all. How can we automate this?
Create a Word2Vec model for a similarity search
Word2Vec was invented by Tomas Mikolov and his colleagues at Google in 2013. It uses neural networks to create models that understand the context of words. There are already trained models for different languages. In our case, we build our model with all the available search queries we have paid for in the past.
Google Ads optimization use case: Find negative search patterns and add them as negative keywords
Let’s assume we have a Google Ads account that contains keywords for all product brands: +hugo +boss.
Using n-gram analysis, we found that some people search for the pattern brand + location. The search intent is to buy locally and not online. This is why cities like “Berlin” and “Hamburg” have high CPOs and low conversions when they’re searched for.
It’s time to query the model with “berlin” and get some similar words:
{“berlin”: [[“münchen”, 0.7318567037582397], [“hamburg”, 0.6991483569145203], [“düsseldorf”, 0.6703126430511475], [“essen”, 0.6388342976570129], [“wien”, 0.6380628347396851], [“österreich”, 0.6259065270423889], [“nürnberg”, 0.6144401431083679], [“germany”, 0.6049420237541199], [“köln”, 0.6002721786499023], [“hannover”, 0.5998085737228394], [“austria”, 0.5866931080818176], [“graz”, 0.5863818526268005], [“stuttgart”, 0.5808620452880859], [“deutschland”, 0.5711857676506042], [“duitsland”, 0.5685932040214539], [“munich”, 0.5610017776489258], [“frankfurt”, 0.5607503652572632], [“dresden”, 0.5480561256408691], [“aachen”, 0.5386894345283508], [“regensburg”, 0.5285172462463379]]}
We got some pretty impressive results. The output shows similar words along with their similarity value. In this case, I limited the results to the top 20.
What does this mean for my negative keyword list? Based on only 1-gram (“berlin”), for which we have enough click data, the model suggests a list of very similar words that currently don’t have enough examples to grab our attention if we only look at the n-gram list.
We use the results to add a lot of new negative keywords that prevent us from paying for future searches that are unlikely to convert.
The whole process now looks like this:
- Classify negative search patterns based on their n-gram data, if we have enough data.
- Use this classification as input to query our word2vec model and get thousands of similar words.
This two-step approach is practical for Google Ads accounts that are using:
- Phrase, Modified Broad, or Broad match keywords
- Google Shopping
- Dynamic Search Ads
Advantages of using word2vec for finding negative keywords:
- Unsupervised learning: Word2vec is an unsupervised learning algorithm, which means it can learn and adapt to new data without human intervention.
- Faster processing: Word2vec can process large amounts of text data quickly and efficiently.
- Scalability: Word2vec can analyze large amounts of text data, making it suitable for extensive keyword research and analysis.
Do you need a developer?
Don’t let technical hurdles keep you from success. If you feel overwhelmed with the technical issues of your project, it’s time to contact us. Our experienced developers can help you avoid hours of frustration and failed attempts. Email us now.